Nonparametric Bayesian Learning for Collaborative Robot Multimodal Introspection
Xuefeng Zhou,Hongmin Wu,Juan Rojas,Zhihao Xu,Shuai Li
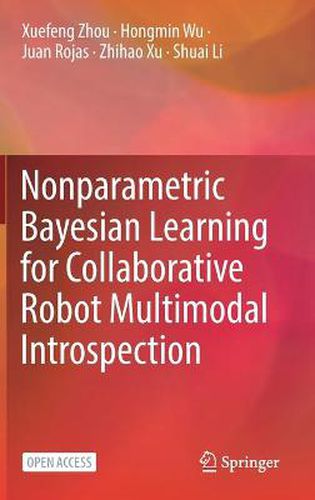
Nonparametric Bayesian Learning for Collaborative Robot Multimodal Introspection
Xuefeng Zhou,Hongmin Wu,Juan Rojas,Zhihao Xu,Shuai Li
This title is printed to order. This book may have been self-published. If so, we cannot guarantee the quality of the content. In the main most books will have gone through the editing process however some may not. We therefore suggest that you be aware of this before ordering this book. If in doubt check either the author or publisher’s details as we are unable to accept any returns unless they are faulty. Please contact us if you have any questions.
This open access book focuses on robot introspection, which has a direct impact on physical human-robot interaction and long-term autonomy, and which can benefit from autonomous anomaly monitoring and diagnosis, as well as anomaly recovery strategies. In robotics, the ability to reason, solve their own anomalies and proactively enrich owned knowledge is a direct way to improve autonomous behaviors. To this end, the authors start by considering the underlying pattern of multimodal observation during robot manipulation, which can effectively be modeled as a parametric hidden Markov model (HMM). They then adopt a nonparametric Bayesian approach in defining a prior using the hierarchical Dirichlet process (HDP) on the standard HMM parameters, known as the Hierarchical Dirichlet Process Hidden Markov Model (HDP-HMM). The HDP-HMM can examine an HMM with an unbounded number of possible states and allows flexibility in the complexity of the learned model and the development of reliable and scalable variational inference methods.
This book is a valuable reference resource for researchers and designers in the field of robot learning and multimodal perception, as well as for senior undergraduate and graduate university students.
This item is not currently in-stock. It can be ordered online and is expected to ship in 7-14 days
Our stock data is updated periodically, and availability may change throughout the day for in-demand items. Please call the relevant shop for the most current stock information. Prices are subject to change without notice.
Sign in or become a Readings Member to add this title to a wishlist.