Image Quality Assessment of Computer-generated Images: Based on Machine Learning and Soft Computing
Andre Bigand,Julien Dehos,Christophe Renaud,Joseph Constantin
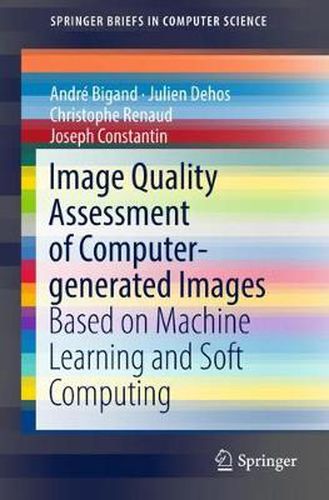
Image Quality Assessment of Computer-generated Images: Based on Machine Learning and Soft Computing
Andre Bigand,Julien Dehos,Christophe Renaud,Joseph Constantin
This title is printed to order. This book may have been self-published. If so, we cannot guarantee the quality of the content. In the main most books will have gone through the editing process however some may not. We therefore suggest that you be aware of this before ordering this book. If in doubt check either the author or publisher’s details as we are unable to accept any returns unless they are faulty. Please contact us if you have any questions.
Image Quality Assessment is well-known for measuring the perceived image degradation of natural scene images but is still an emerging topic for computer-generated images. This book addresses this problem and presents recent advances based on soft computing. It is aimed at students, practitioners and researchers in the field of image processing and related areas such as computer graphics and visualization.
In this book, we first clarify the differences between natural scene images and computer-generated images, and address the problem of Image Quality Assessment (IQA) by focusing on the visual perception of noise. Rather than using known perceptual models, we first investigate the use of soft computing approaches, classically used in Artificial Intelligence, as full-reference and reduced-reference metrics. Thus, by creating Learning Machines, such as SVMs and RVMs, we can assess the perceptual quality of a computer-generated image. We also investigate the use of interval-valued fuzzy sets as a no-reference metric.
These approaches are treated both theoretically and practically, for the complete process of IQA. The learning step is performed using a database built from experiments with human users and the resulting models can be used for any image computed with a stochastic rendering algorithm. This can be useful for detecting the visual convergence of the different parts of an image during the rendering process, and thus to optimize the computation. These models can also be extended to other applications that handle complex models, in the fields of signal processing and image processing.
This item is not currently in-stock. It can be ordered online and is expected to ship in 7-14 days
Our stock data is updated periodically, and availability may change throughout the day for in-demand items. Please call the relevant shop for the most current stock information. Prices are subject to change without notice.
Sign in or become a Readings Member to add this title to a wishlist.