Bayesian Inference for Probabilistic Risk Assessment: A Practitioner's Guidebook
Dana Kelly,Curtis Smith
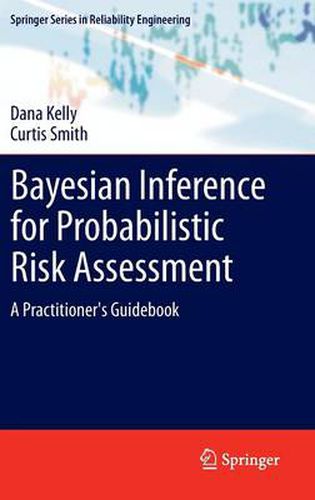
Bayesian Inference for Probabilistic Risk Assessment: A Practitioner’s Guidebook
Dana Kelly,Curtis Smith
This title is printed to order. This book may have been self-published. If so, we cannot guarantee the quality of the content. In the main most books will have gone through the editing process however some may not. We therefore suggest that you be aware of this before ordering this book. If in doubt check either the author or publisher’s details as we are unable to accept any returns unless they are faulty. Please contact us if you have any questions.
Bayesian Inference for Probabilistic Risk Assessment provides a Bayesian foundation for framing probabilistic problems and performing inference on these problems. Inference in the book employs a modern computational approach known as Markov chain Monte Carlo (MCMC). The MCMC approach may be implemented using custom-written routines or existing general purpose commercial or open-source software. This book uses an open-source program called OpenBUGS (commonly referred to as WinBUGS) to solve the inference problems that are described. A powerful feature of OpenBUGS is its automatic selection of an appropriate MCMC sampling scheme for a given problem. The authors provide analysis building blocks that can be modified, combined, or used as-is to solve a variety of challenging problems.
The MCMC approach used is implemented via textual scripts similar to a macro-type programming language. Accompanying most scripts is a graphical Bayesian network illustrating the elements of the script and the overall inference problem being solved. Bayesian Inference for Probabilistic Risk Assessment also covers the important topics of MCMC convergence and Bayesian model checking.
Bayesian Inference for Probabilistic Risk Assessment is aimed at scientists and engineers who perform or review risk analyses. It provides an analytical structure for combining data and information from various sources to generate estimates of the parameters of uncertainty distributions used in risk and reliability models.
This item is not currently in-stock. It can be ordered online and is expected to ship in 7-14 days
Our stock data is updated periodically, and availability may change throughout the day for in-demand items. Please call the relevant shop for the most current stock information. Prices are subject to change without notice.
Sign in or become a Readings Member to add this title to a wishlist.