Interactive Imitation Learning in Robotics
Carlos Celemin, Rodrigo Perez-Dattari, Eugenio Chisari, Giovanni Franzese, Leandro de Souza Rosa, Ravi Prakash, Zlatan Ajanovic, Marta Ferraz, Abhinav Valada, Jens Kober
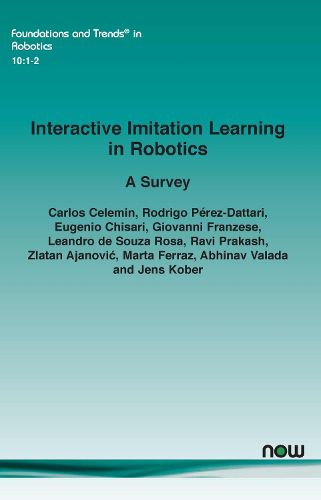
Interactive Imitation Learning in Robotics
Carlos Celemin, Rodrigo Perez-Dattari, Eugenio Chisari, Giovanni Franzese, Leandro de Souza Rosa, Ravi Prakash, Zlatan Ajanovic, Marta Ferraz, Abhinav Valada, Jens Kober
This title is printed to order. This book may have been self-published. If so, we cannot guarantee the quality of the content. In the main most books will have gone through the editing process however some may not. We therefore suggest that you be aware of this before ordering this book. If in doubt check either the author or publisher’s details as we are unable to accept any returns unless they are faulty. Please contact us if you have any questions.
Existing robotics technology is still mostly limited to being used by expert programmers who can adapt the systems to new required conditions, but not flexible and adaptable by non-expert workers or end-users. Imitation Learning (IL) has obtained considerable attention as a potential direction for enabling all kinds of users to easily program the behavior of robots or virtual agents. Interactive Imitation Learning (IIL) is a branch of Imitation Learning (IL) where human feedback is provided intermittently during robot execution allowing an online improvement of the robot's behavior.
In this monograph, research in IIL is presented and low entry barriers for new practitioners are facilitated by providing a survey of the field that unifies and structures it. In addition, awareness of its potential is raised, what has been accomplished and what are still open research questions being covered.
Highlighted are the most relevant works in IIL in terms of human-robot interaction (i.e., types of feedback), interfaces (i.e., means of providing feedback), learning (i.e., models learned from feedback and function approximators), user experience (i.e., human perception about the learning process), applications, and benchmarks. Furthermore, similarities and differences between IIL and Reinforcement Learning (RL) are analyzed, providing a discussion on how the concepts offline, online, off-policy and on-policy learning should be transferred to IIL from the RL literature.
Particular focus is given to robotic applications in the real world and their implications are discussed, and limitations and promising future areas of research are provided.
This item is not currently in-stock. It can be ordered online and is expected to ship in 7-14 days
Our stock data is updated periodically, and availability may change throughout the day for in-demand items. Please call the relevant shop for the most current stock information. Prices are subject to change without notice.
Sign in or become a Readings Member to add this title to a wishlist.