Identifying Bearing Rotordynamic Coefficients Using an Extended Kalman Filter
Bard a Miller,Samuel a Howard
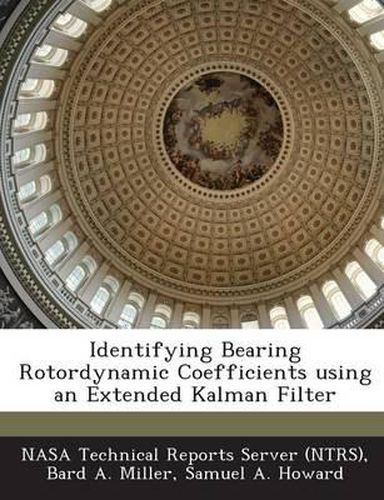
Identifying Bearing Rotordynamic Coefficients Using an Extended Kalman Filter
Bard a Miller,Samuel a Howard
An Extended Kalman Filter is developed to estimate the linearized direct and indirect stiffness and damping force coefficients for bearings in rotor-dynamic applications from noisy measurements of the shaft displacement in response to imbalance and impact excitation. The bearing properties are modeled as stochastic random variables using a Gauss-Markov model. Noise terms are introduced into the system model to account for all of the estimation error, including modeling errors and uncertainties and the propagation of measurement errors into the parameter estimates. The system model contains two user-defined parameters that can be tuned to improve the filter s performance; these parameters correspond to the covariance of the system and measurement noise variables. The filter is also strongly influenced by the initial values of the states and the error covariance matrix. The filter is demonstrated using numerically simulated data for a rotor-bearing system with two identical bearings, which reduces the number of unknown linear dynamic coefficients to eight. The filter estimates for the direct damping coefficients and all four stiffness coefficients correlated well with actual values, whereas the estimates for the cross-coupled damping coefficients were the least accurate.
This item is not currently in-stock. It can be ordered online and is expected to ship in approx 2 weeks
Our stock data is updated periodically, and availability may change throughout the day for in-demand items. Please call the relevant shop for the most current stock information. Prices are subject to change without notice.
Sign in or become a Readings Member to add this title to a wishlist.