Machine Learning for Powder-Based Metal Additive Manufacturing
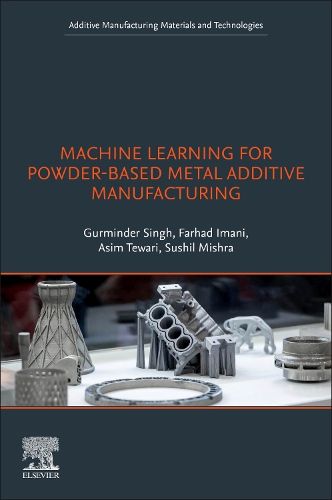
Machine Learning for Powder-Based Metal Additive Manufacturing
Machine Learning for Powder-based Metal Additive Manufacturing outlines machine learning (ML) methods for additive manufacturing (AM) of metals that will improve product quality, optimize manufacturing processes, and reduce costs. The book combines ML and AM methods to develop intelligent models that train AM techniques in pre-processing, process optimization, and post-processing for optimized microstructure, tensile and fatigue properties, and biocompatibility for various applications. The book covers ML for design in AM, ML for materials development and intelligent monitoring in metal AM, both geometrical deviation and physics informed machine learning modeling, as well as data-driven cost estimation by ML. In addition, optimization for slicing and orientation, ML to create models of materials for AM processes, ML prediction for better mechanical and microstructure prediction, and feature extraction by sensing data are all covered, and each chapter includes a case study.
Order online and we’ll ship when available (1 November 2024)
Our stock data is updated periodically, and availability may change throughout the day for in-demand items. Please call the relevant shop for the most current stock information. Prices are subject to change without notice.
Sign in or become a Readings Member to add this title to a wishlist.