Unified Methods for Censored Longitudinal Data and Causality
Mark J. van der Laan,James M Robins
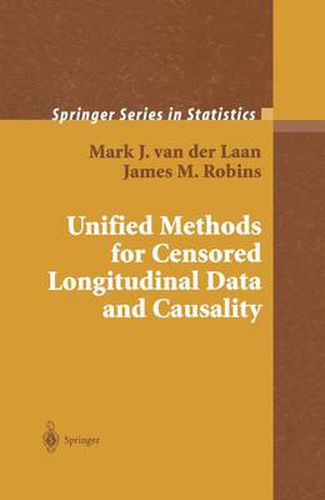
Unified Methods for Censored Longitudinal Data and Causality
Mark J. van der Laan,James M Robins
This title is printed to order. This book may have been self-published. If so, we cannot guarantee the quality of the content. In the main most books will have gone through the editing process however some may not. We therefore suggest that you be aware of this before ordering this book. If in doubt check either the author or publisher’s details as we are unable to accept any returns unless they are faulty. Please contact us if you have any questions.
During the last decades, there has been an explosion in computation and information technology. This development comes with an expansion of complex observational studies and clinical trials in a variety of fields such as medicine, biology, epidemiology, sociology, and economics among many others, which involve collection of large amounts of data on subjects or organisms over time. The goal of such studies can be formulated as estimation of a finite dimensional parameter of the population distribution corresponding to the observed time- dependent process. Such estimation problems arise in survival analysis, causal inference and regression analysis. This book provides a fundamental statistical framework for the analysis of complex longitudinal data. It provides the first comprehensive description of optimal estimation techniques based on time-dependent data structures subject to informative censoring and treatment assignment in so called semiparametric models. Semiparametric models are particularly attractive since they allow the presence of large unmodeled nuisance parameters. These techniques include estimation of regression parameters in the familiar (multivariate) generalized linear regression and multiplicative intensity models. They go beyond standard statistical approaches by incorporating all the observed data to allow for informative censoring, to obtain maximal efficiency, and by developing estimators of causal effects. It can be used to teach masters and Ph.D. students in biostatistics and statistics and is suitable for researchers in statistics with a strong interest in the analysis of complex longitudinal data.
This item is not currently in-stock. It can be ordered online and is expected to ship in 7-14 days
Our stock data is updated periodically, and availability may change throughout the day for in-demand items. Please call the relevant shop for the most current stock information. Prices are subject to change without notice.
Sign in or become a Readings Member to add this title to a wishlist.